Introduction:
In today’s rapidly evolving pharmaceutical and medical device industries, maintaining high standards of quality assurance is paramount. As technology continues to advance, innovative solutions such as artificial intelligence (AI) and automation are revolutionizing the validation process. Artificial intelligence (AI) is rapidly transforming the world around us. From virtual assistants to self-driving cars, AI has permeated many aspects of our lives. One such area where AI is, can, and will be making a significant impact is in the quality field. By harnessing the power of AI in quality management, businesses can identify and address quality issues before they escalate, ensuring that products and services meet customer expectations.
In this new era of Digital Transformation, Quality is no longer about raw data, but the way we process the data and the insights we extract from it. There is no doubt that the combination of Artificial Intelligence (AI) and Quality Management is not just a dream but is already reshaping the way we do business today. It’s a game-changer.
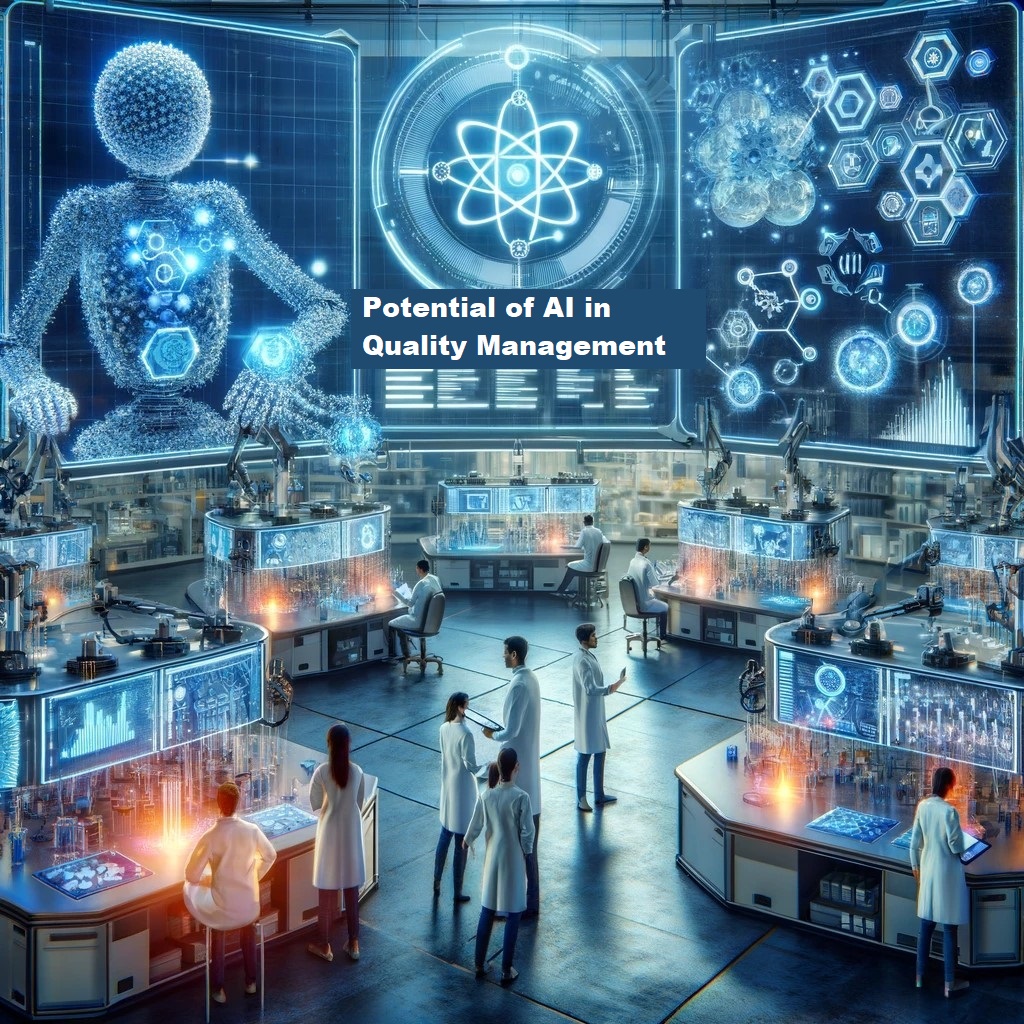
AI's Role in Transforming Traditional Quality Management Systems
AI is revolutionizing the way we work, offering a pathway to streamlined operations, smarter decisions, and higher customer satisfaction for Quality professionals. It brings a suite of benefits including automation, early problem detection, ongoing enhancement, instantaneous decision-making, and better use of resources. This marks a significant leap forward in how we approach and manage quality in our industries. Let’s delve into how these advantages can transform our professional landscape.
When AI and QMS come together, they create a powerful synergy that enhances Quality control and optimization.
1. Data-Driven Decision-Making
2. Automated Quality Control
3. Predictive Quality Management:
Role of AI in Data-Driven Decision Making:
AI is poised to revolutionize data-driven decision-making in the pharmaceutical industry by providing advanced analytics, predictive modeling, and actionable insights. Here’s how AI is set to transform decision-making processes, along with two compelling example.
AI-powered predictive analytics algorithms can analyze vast amounts of historical data to identify patterns, trends, and correlations. By leveraging this capability, pharmaceutical companies can anticipate future outcomes and make informed decisions based on data-driven insights.
Example :
- Drug Discovery and Development: In drug discovery and development, AI algorithms can analyze molecular structures, biological pathways, and clinical trial data to predict the efficacy and safety of potential drug candidates. By identifying promising drug candidates early in the development process, pharmaceutical companies can allocate resources more efficiently and prioritize investments in the most promising therapies.
- Personalized Medicine: AI enables personalized medicine by analyzing patient data, including genetic information, medical history, and lifestyle factors, to tailor treatment plans to individual patients’ needs. This approach allows healthcare providers to deliver more targeted and effective treatments, improving patient outcomes and reducing healthcare costs.
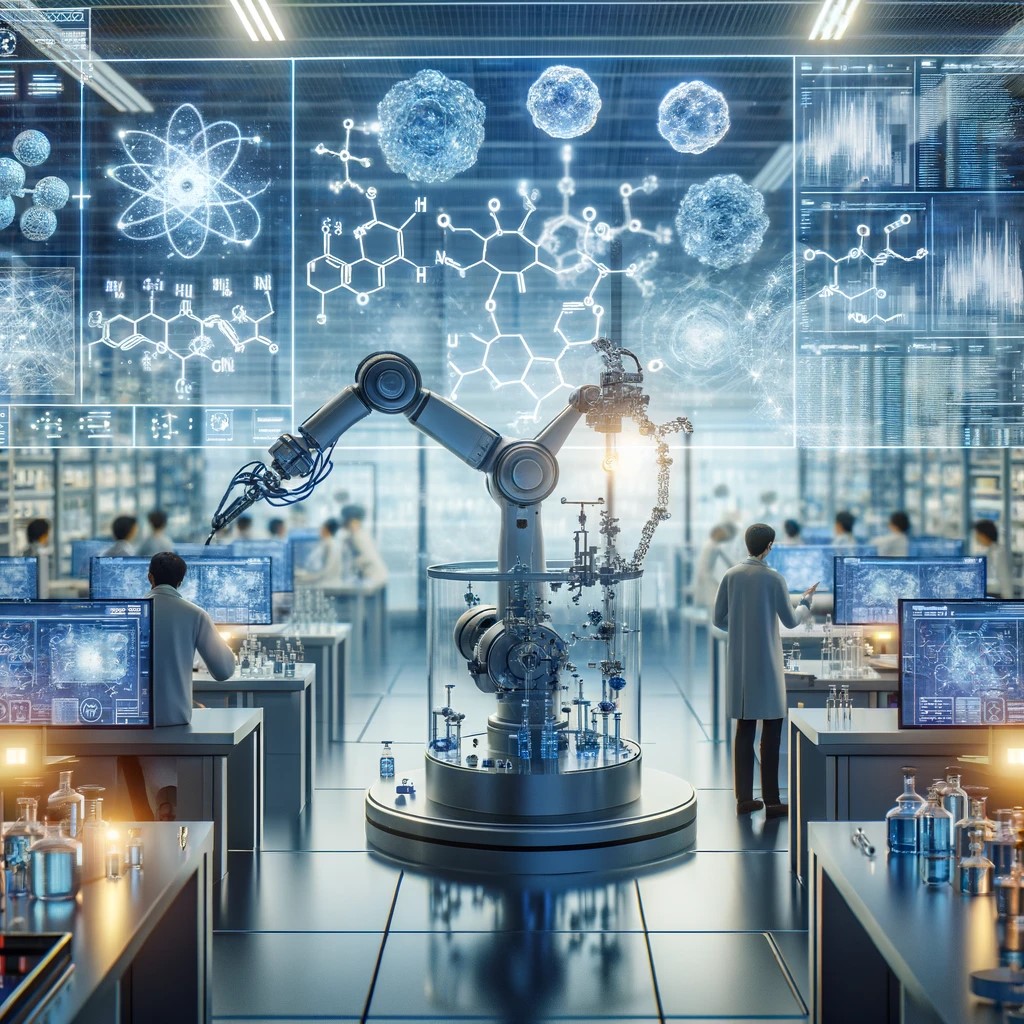
Role of AI in Automated Quality Control:
AI holds tremendous potential for transforming automated quality control in the pharmaceutical industry by enhancing data analysis, predictive maintenance, process optimization, quality assurance automation, and regulatory compliance. As AI technologies continue to evolve and mature, they will play an increasingly critical role in ensuring product quality, safety, and efficacy in pharmaceutical manufacturing.
Explore how AI enhances quality processes with a real-world example.
How AI Can Help in below situation, lets understand:
A pharmaceutical company is manufacturing tablets in a large-scale production facility. Ensuring the quality of each tablet is crucial to meet regulatory standards and maintain product efficacy and safety.
- Data Analysis and Pattern Recognition: AI analyzes manufacturing data, detecting deviations and patterns, indicating potential quality issues. For instance, it identifies subtle variations in tablet weight, signalling manufacturing process problems.
- Anomaly Detection and Fault Identification: AI-driven systems enable real-time production monitoring, identifying deviations promptly. Employing machine learning, AI distinguishes normal variations from anomalies. For example, detecting sudden temperature spikes in tablet compression alerts to potential equipment issues.
- Predictive Maintenance and Process Optimization: AI-driven predictive analytics anticipates equipment failures, enabling proactive maintenance. By analyzing sensor data, AI detects early warning signs, like subtle vibration changes, prompting timely interventions. Example: AI predicts tablet press malfunction, guiding proactive inspection.
- Quality Assurance Feedback Loop: AI enhances quality management by offering real-time feedback on product quality and process performance. It correlates metrics with production parameters to pinpoint root causes of issues, aiding in timely corrective actions. For example, if tablets fail dissolution testing, AI analyzes process data to identify manufacturing stage issues, empowering process engineers for adjustments.
Role of AI in Predictive Quality Management:
AI transforms predictive quality management in pharma with advanced analytics, proactively identifying risks. Discover how AI enables proactive quality control with a real-world example.
Use Case: Predicting Manufacturing Defects with AI
Example: A pharmaceutical company manufactures a critical drug product in high volumes. Despite rigorous quality control measures, occasional manufacturing defects still occur, leading to costly recalls and delays.
How AI Can Help:
- Enhanced Data Integration: AI integrates data from various sources like equipment sensors, QC systems, and historical data, providing insights into production and quality trends.
- Predictive Analytics: AI uses machine learning to analyze historical data, predicting potential defects.
- Real-time Monitoring: AI continuously monitors manufacturing data, detecting anomalies for proactive intervention.
- Root Cause Analysis: AI conducts thorough root cause analysis to prevent recurrence.
Challenges of integrating AI into quality management
The effectiveness of AI in quality management heavily depends on the quality and accuracy of data. Organizations must prioritize data collection, cleansing, and normalization to feed AI systems with reliable data.
Successful AI implementation requires a team with a strong understanding of AI and data science. Investing in training and upskilling is essential to bridge the skills gap and fully utilize AI capabilities
Ensuring the privacy and security of data used in AI systems is non-negotiable. Businesses must adhere to privacy laws and implement stringent security protocols to protect sensitive information
Integrating AI technologies with existing quality management systems can be challenging. Compatibility and interoperability issues must be addressed to ensure seamless operation.
The initial investment and ongoing costs for AI implementation can be significant. Organizations need to carefully plan resource allocation to support the technology without compromising other operational areas.
Strategies for Successfully Integrating AI Into Existing Quality Management Workflows
Integrating artificial intelligence (AI) into existing quality management workflows presents significant opportunities for efficiency and innovation in the pharmaceutical and medical device industry. By strategically implementing AI-driven solutions, companies can enhance quality assurance processes, improve decision-making, and ensure regulatory compliance.
Let’s delve into a real-time example showcasing how AI-powered quality control is revolutionizing pharmaceutical manufacturing.
Example: AI-Powered Image Recognition in Tablet Inspection
Scenario: A pharmaceutical manufacturing company produces a range of tablets for various medical purposes. Ensuring the quality of these tablets is crucial to meet regulatory standards and maintain patient safety. However, manual inspection processes are time-consuming and prone to human error, leading to inefficiencies and potential quality issues.
Solution:
To address these challenges, the company implements an AI-powered image recognition system for tablet inspection. This system utilizes advanced machine learning algorithms to analyze images of tablets captured during the manufacturing process.
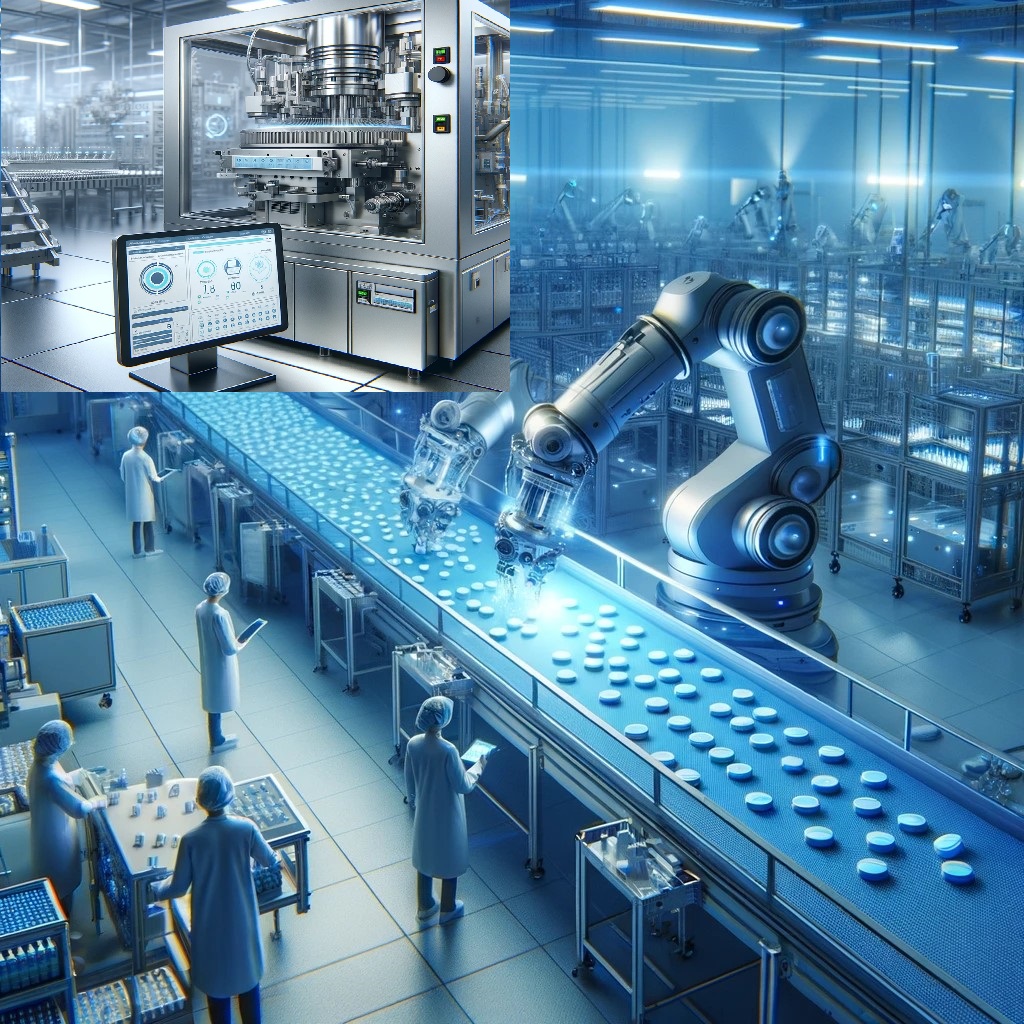
Implementation Steps to apply solution:
Data Collection: The company gathers a diverse dataset of tablet images, including both acceptable and defective samples. These images represent various characteristics such as size, shape, color, and surface defects
Model Development: Data scientists create a powerful AI tool called a convolutional neural network (CNN) to spot quality issues in tablets. This smart system learns from data to tell good from bad pills by their appearance
Integration with Manufacturing Process: The AI-powered image recognition system is integrated into the manufacturing line, where cameras capture images of tablets as they pass through inspection points. The system analyzes these images in real-time, flagging any tablets that deviate from predefined quality criteria.
Validation and Testing: Before deployment, the AI model undergoes rigorous validation and testing to ensure its accuracy and reliability. This includes comparing its performance against human inspectors and evaluating its ability to detect various types of defects.
Conclusion:
Merging AI with existing quality management in pharma and medical devices spells big wins: efficiency up, accuracy better, and compliance on point. It’s all about smart strategy and learning from those who’ve done it, to let AI boost innovation and top-notch quality assurance.
Enter AI and automation, changing the game in life sciences quality assurance. These tech wonders work magic on data analysis, risk handling, and making processes smooth. They’re our allies in spotting and fixing quality snags early, making sure compliance is spotless, and making our workflow slick.
From our corner as quality and regulatory advisors, we’re watching a revolution unfold. AI and automation are not just changing the game; they’re setting a new high score for product quality and safety for patients. In the fast-paced world of life sciences, jumping on the AI bandwagon isn’t just smart; it’s essential for staying ahead.
Useful Link:
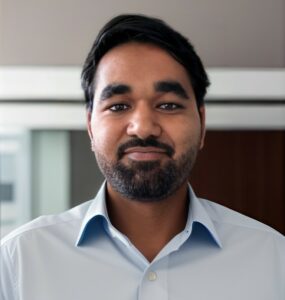
Sagar Pawar
Computer System Validation Specialist